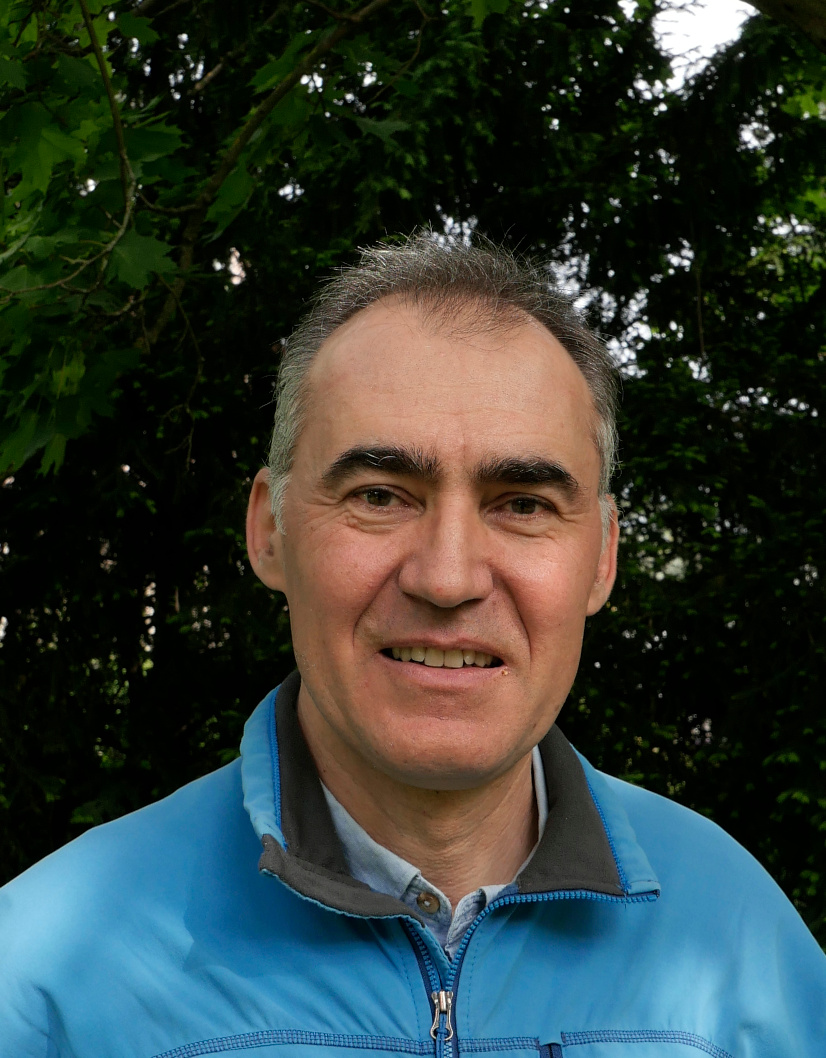
Stefan Leutgeb, Ph.D.
Professor of Neurobiology
University of California San Diego
Born in 1969 in Wels, Austria
Studied Biology at Paris Lodron University Salzburg, Psychology at Bowling Green State University, and Neuroscience at the University of Utah
Project
Neural Computations in Real Brains and in Artificial Systems
Although neural computations are the cornerstone of both neuroscience and artificial intelligence, the biological foundations of how large populations of neurons perform computations in the brain are only beginning to be revealed. Among the approaches to identify the underpinnings of neural computations, the brain’s navigation system provides particularly intriguing access. Although brain regions that support navigation, such as the hippocampus and entorhinal cortex, are removed from sensory inputs and motor outputs by multiple processing stages, neuronal activity patterns emerge in these brain regions that correspond to readily identifiable physical locations in the real world. Collectively, the activity patterns of single neurons therefore form a map-like representation of the natural world. Although moderately complex computational models can readily reproduce this phenomenon, it remains unclear how closely the models capture the key computations in a critical set of connections between neurons that perform these computations in real brains. The work that is proposed here will first review successful case studies – including from invertebrate models – that have identified brain circuits for spatial navigation and underlying computations. We will then take a comprehensive approach to ask whether these studies can be extrapolated to increasingly more complex systems or whether we need to rethink how neural computations can be identified once large populations of neurons are connected across multiple brain regions. Although our thinking will be grounded in biological systems, it will also consider approaches from a broad range of disciplines, such as the visual arts, social sciences (e.g., geography), economics, and computer science.Recommended Reading
Leutgeb, Stefan, Jill K. Leutgeb, Carol A. Barnes, Edvard I. Moser, Bruce L. McNaughton, and May-Britt Moser (2005). “Independent Codes for Spatial and Episodic Memory in Hippocampal Neuronal Ensembles.” Science 309: 619–623. https://doi.org/10.1126/science.1114037.
Koenig, Julie, Ashley N. Linder, Jill K. Leutgeb, and Stefan Leutgeb (2011). “The Spatial Periodicity of Grid Cells Is Not Sustained during Reduced Theta Oscillations.” Science 332: 592–595. https://doi.org/10.1126/science.1201685.
Quirk, Clare R., Ipshita Zutshi, Sunandha Srikanth, Maylin L. Fu, Naomie Devico Marciano, Morgan K. Wright, Darian F. Parsey, et al. (2021). “Precisely Timed Theta Oscillations Are Selectively Required during the Encoding Phase of Memory.” Nature Neuroscience 24: 1614–1627. https://doi.org/10.1038/s41593-021-00919-0.
Colloquium, 31.10.2023
The Brain’s Positioning System and its Simulation in Artificial Neural Networks
Getting from point A to point B and navigating in complex environments is now often performed with the help of specialized software and devices, but it is of course a cognitive function that has for millions of years been essential to the survival of any animal species, including humans. Not surprisingly, these computations are performed with ease by organisms that have a wide range of brain size and complexity, but how? We will start with a look at the building blocks of the brain and at simple wiring diagrams, which are also the template for artificial neural networks. With an understanding of the basic components, we will then take a brief look at the question of brain size and at the extent to which neural networks can be scaled up by just adding more brain cells. In this regard, it is interesting to consider that the ever-increasing power of artificial intelligence is not arising from much added sophistication, but predominantly from expanding network size. This leads us to the question whether added complexity in the brain, not only in numbers, but also in network architecture is critical for intelligence. The brain circuits that support navigation are exemplary for these considerations because they are at the top of hierarchically stacked layers – many levels removed from sensory input and from motor output. This could lead us to the assumption that we may not understand much from directly investigating the top layer, but that we should first understand neural computations at the intermediary stages. However, even without understanding the computations that are performed at the intermediary stages, we can make a number of surprisingly insightful observations in what we might consider the most complex layers in these systems. For example, we can identify single brain cells that are highly active when animals are pointing their head in a particular direction or others that are highly active when animals occupy a particular location in space. In addition, there are even more abstract activity patterns, such as those that seemingly correspond to grid lines on a map. The latter finding is particularly remarkable, because it allows us to directly observe a cognitive construct for which there is no obvious direct correspondence in the environment – at least not until cartographers devised the use of coordinate systems. Despite these glimpses into the brain, there remains a large gap in our understanding of the brain’s computations and whether they are powered by numbers, by anatomical organization, or by emergent activity patterns. Furthermore, if we were to better understand the brain’s computations, could we use these insights to make artificial systems even more intelligent? Some of these questions may be answered at the interface of neuroscience and computer science, and navigation systems could be particularly useful for providing key insights.
Publications from the Fellows' Library
Leutgeb, Stefan (New York, NY, 2021)
Precisely timed theta oscillations are selectively required during the encoding phase of memory
Leutgeb, Stefan (New York, NY Nature America, 2018)
Leutgeb, Stefan (Washington, DC, 2012)
Neuronal code for extended time in the hippocampus
Leutgeb, Stefan (Washington, DC, 2007)
Pattern separation in the dentate gyrus and CA3 of the hippocampus