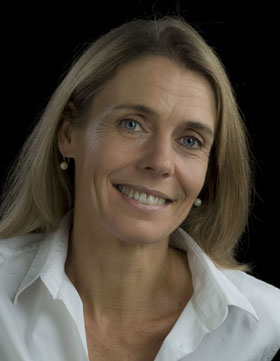
Systems Theory and Algorithmic Futures
Interview with Elena Esposito
Elena Esposito is Professor of Sociology at Bielefeld University, Germany, and at the University of Bologna, Italy. She studied philosophy with Umberto Eco and earned her doctorate in sociology with Niklas Luhmann. Her glossary on Niklas Luhmann’s theory of social systems (1997) has become an introductory reference book for sociological-systems theory. Her current research project, “The Future of Prediction: The Social Consequences of Algorithmic Forecast in Insurance, Medicine and Policing” is supported by a five-year Advanced Grant from the European Research Council. In 2019/2020 she was a Fellow at the Wissenschaftskolleg zu Berlin.
Excerpt of an interview conducted by Katrin Sold and Bénédicte Zimmermann. The full interview has been published under the title “Systems Theory and Algorithmic Futures: Interview with Elena Esposito” in the journal Constructivist Foundations (Vol. 16, no. 3, 356-361).
Interviewer: How does systems theory bind together the past, the present and the future?
EE: Luhmann developed his idea of time as the temporalization of complexity, referring to Koselleck’s concept of “futures past” (Koselleck 2004). Time starts from a present that immediately disappears and refers to a future not yet arrived and a past which is no more. These different horizons of time are reflexive because in both the past and future there are other presents with their own past and future horizons. There are horizons within horizons. Our present, for example, belongs to the future of a past present. And in our present we refer to future presents, for which our present will be in the past. Our present decisions can influence how this future present will look. This intertwining of temporal horizons is a striking example of open possibilities or contingency. Things can be and could have always been different. The possibilities are open-ended but they depend on each other. What will be possible in the future depends also on what we do today, and this as a consequence of past events.
The specificity of systems theory, in my view, is to connect the concept of time to social structures. Like other concepts, the concept of time itself changes over time, and this process is closely linked with the evolution of society. In the ancient world the idea of time was very different from ours, which is a typically modern idea. In the past, time was not structured along the distinction between past and future but along the distinction between eternity and tempus – with eternity referring to the time of God and tempus to the (limited) time of human beings. From the point of view of systems theory as a theory of society, with the dawn of modernity and the evolution of a differentiated society the idea of time changed progressively, developing this kind of reflexive structure of past, present and future that we observe today. So the sociological question is: Why?
To answer this question, systems theory refers again to the structure of society. The relatively simple dualism of eternity and tempus corresponds to a rather traditional society understood as a cosmos of order. This order is the same for every human being throughout his and her time on earth – tempus – and remains stable. But when society became more complex through functional differentiation, the temporal structure of tempus and eternity was not complex enough. Things changed too quickly during people’s lifetime, which led to a redefinition of the distinction between the past, the present and the future. The contribution of systems theory is to highlight this relationship between the semantic structure of time and the state of society.
Interviewer: As you underscore it, time raises the issue of the past as well as the future. How exactly does systems theory address the future?
EE: First of all we have to remember that the future does not yet exist. The future is uncertain; it is produced by our decisions. We therefore have to distinguish between the present future and the future present. The present future is the future as we can imagine it now, according to our information and current probabilities. We develop future scenarios on the basis of what we know now and take decisions according to what we expect. That which will become real in the future – we call this the future present – can be very different from these scenarios, especially because our plans can change the shape of that future. Due to our decisions the future present can become different from the future that we now imagine. A very interesting example is how finance deals with the future. Finance has all sorts of plans and predictions that very often are disappointed, and the failure is also due to the fact that they have been followed, thereby changing the conditions of the future they were expected to predict. Sociologists ever since Merton have acknowledged these dynamics of self-fulfilling and self-defeating prophecies, but the performativity debate helped clarify it even further (e.g. MacKenzie 2006). I myself elaborated these topics in my work on the temporality of finance (Esposito 2011).
Interviewer: Prediction is one way of dealing with the uncertainty of the future. In your current European Research Council (ERC) research project you address new forms of prediction through algorithms. What is the difference between probabilistic and algorithmic predictions?
EE: The relation between probabilistic and algorithmic forms of prediction is a huge topic. In the ERC project we try to focus on the sociological aspect of this relationship and particularly on what we call shared uncertainty. Nobody can know the future in advance, it is uncertain for everyone. But a probabilistic calculus offers tools to face the uncertainty of the future in a controlled way, in the form of forecasts referring to average values. One example of the model of shared uncertainty is the insurance system. Since nobody can predict the future, we all share the same uncertainty about possible future damage, so we are willing to pay a little insurance premium calculated on average future probabilities. For most of us this will be to little purpose, but as we do not know who will be hit by an accident everybody wants to be protected. A similar phenomenon can be observed in other spheres of our society, like medicine or policing, which together with insurance are the ERC project’s three fields of research. Nobody knows in advance who will be sick or who will commit a crime, so everybody is mobilized to cope with this uncertainty.
Different from probabilistic calculus, algorithmic forms of prediction promise to deliver individual predictions. Instead of predicting what will happen in general for an average group of persons, they predict – rightly or wrongly – what will happen to individual persons. The results are still uncertain, but the central question is: How does the use of these predictive tools affect the structure of a society that until then relied on shared uncertainty? Going back to the example of insurance: If we knew precisely who will have a car accident or a health problem, why should everybody pay an insurance premium? The mutualization of risk, which is the basis of the insurance system, would be profoundly endangered. I want to study how these different ways of dealing with uncertainty affect society in the long term.
Interviewer: In your work you draw a parallel between algorithmic techniques and divination (Esposito 2020). What is the rationale for such a parallel?
EE: There is a fascinating parallel between the way algorithmic predictions are constructed today and the way divinatory predictions were constructed in ancient times. In the intervening epoch people used probabilistic calculus and averages to deal with the uncertainty of the future. Probabilistic calculus is connected with a scientific method that can explain phenomena – and thereby project what will happen in the future. Algorithms follow a different procedure: they predict without explaining. By referring to correlations and patterns, they allow for individual predictions of the future. This is technically a return to some of the main features of divinatory forms of prediction, which were discredited when probabilistic calculus was established. Divinatory forms of prediction also refer to correlations and patterns, predict individual events instead of averages, and claim certainty instead of managing uncertainty. In both cases we are dealing with procedures (oracular responses and new “deep learning” techniques) that are basically nontransparent for human beings.
The rise of algorithmic predictions tells us a lot about the relationship between science and non-science. Probabilistic calculus is the basis of many scientific procedures, whereas divination is considered non-scientific – like magic and other forms of superstition. However, scientific methods produced the statistical and informatics tools that led to algorithms – and now algorithms seem to be returning to non-scientific divinatory practices. This relates to a debate triggered by a famous and very controversial article by Chris Anderson in 2008 called “The End of Theory,” claiming that in this time of algorithms science no longer needs theory – it needs neither explanations nor causality. Correlations are enough. It is sufficient to see the what instead of asking why. For example, according to this approach, if you examine the genome you can find patterns and predict illnesses; if the results are reliable you do not have to explain why. There is no time to explain the why, you trust the what and deal with it. This perspective on science is oversimplified and highly debatable, of course, but the development of algorithms challenges basic principles of science such as theory, causation and explanation. I want to explore why tools like algorithms, developed with scientific procedures, lead to the questioning of scientific methods and principles.
References
Esposito, Elena (2020), “Digitale Prognose. Von statistischer Ungewissheit zur algorithmischen Vorhersage,” forthcoming in Alfons Labisch (ed.) Kann Wissenschaft in die Zukunft sehen? Prognosen in den Wissenschaften, Halle, Acta Historica Leopoldina.
Esposito, Elena (2011), The Future of Futures: The Time of Money in Financing and Society, Cheltenham, Edward Elgar.
Koselleck, Reinhart (2004), Futures Past: On the Semantics of Historical Time, New York, Columbia University Press.
MacKenzie, Donald (2006), An Engine, Not a Camera: How Financial Models Shape Markets, Cambridge (Mass.), The MIT Press.